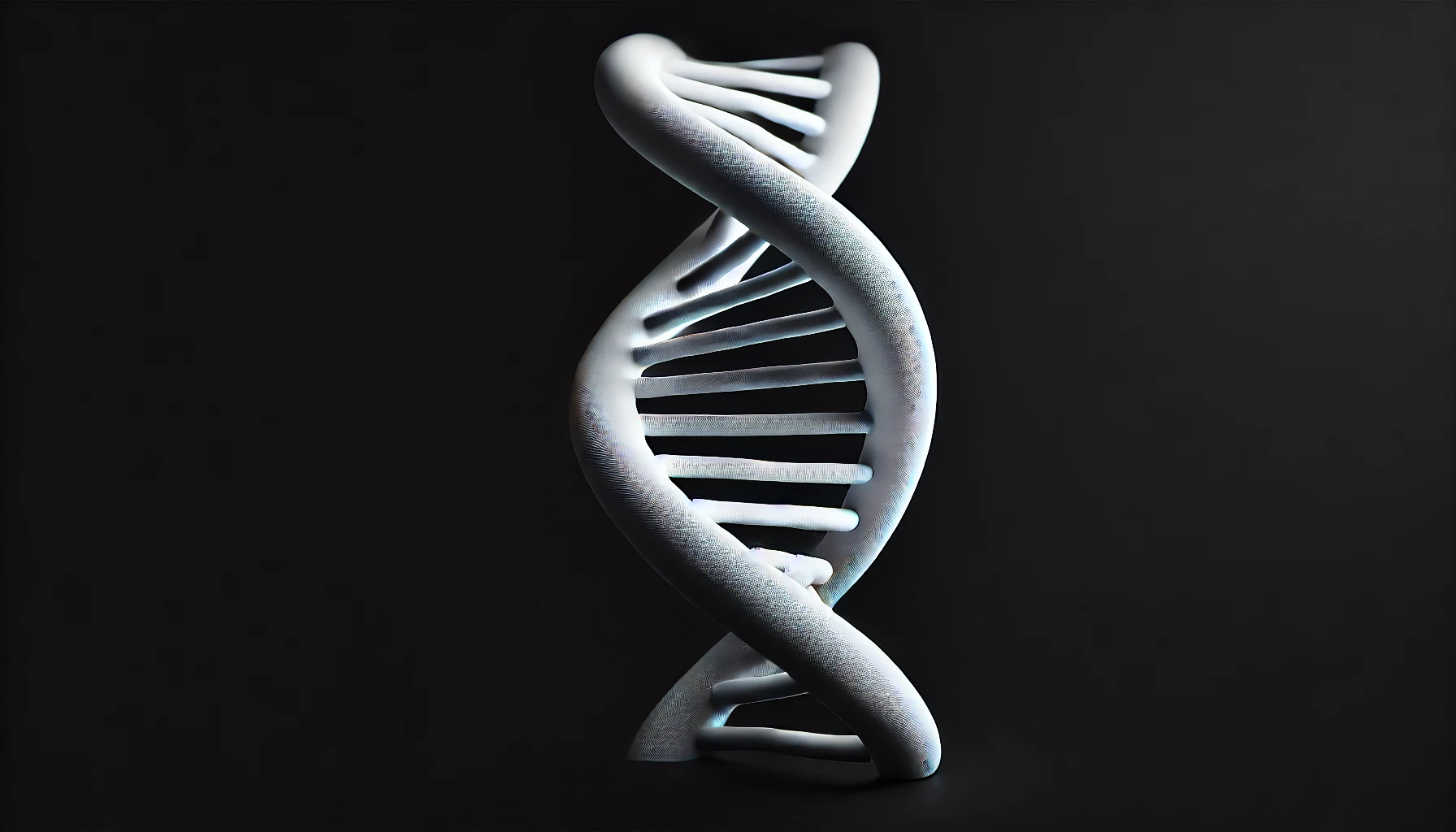
Transformers in Biotech (I) – How it Started Transformer models have emerged as a powerful tool in various domains of…
neuralgap.io
A Quick update: Our Multi-Unimodal Model Paper has been published on ISBI 2025!
Our Paper on our Multi-Unimodal Model architecture has been accepted in the 2025 ISBI conference., and scheduled to be presented in Houston Texas, April 2025!
We hope to see you there!
Interactive image may take a moment to download
Target Identification & Validation
Interactive image may take a moment to download
Hit Discovery & Optimization
Interactive image may take a moment to download
Lead Optimization & Candidate Selection
Transformers in Biotech (I) – How it Started Transformer models have emerged as a powerful tool in various domains of…
©2023. Neuralgap.io